
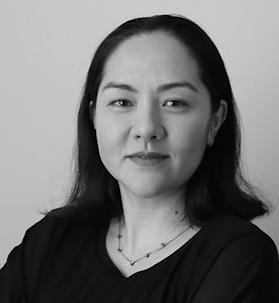
Emille E. O. Ishida, PhD
I am a Brazilian physicist based in France and working in Astronomy and Cosmology since I can remember.
​
My research is focused on machine learning applications to astronomy and in the development of sustainable interdisciplinary scientific environments.
​
I am co-founder of the Cosmostatistics Initiative (COIN),
MY LATEST RESEARCH
Real-Time Active Learning for optimised spectroscopic follow-up: Enhancing early SN Ia classification with the Fink broker
Moller, Ishida et al., 2025
Current and future surveys rely on machine learning classification to obtain large and complete samples of transients. Many of these algorithms are restricted by training samples that contain a limited number of spectroscopically confirmed events. Here, we present the first real-time application of Active Learning to optimise spectroscopic follow-up with the goal of improving training sets of early type Ia supernovae (SNe Ia) classifiers. Using a photometric classifier for early SN Ia, we apply an Active Learning strategy for follow-up optimisation using the real-time FINK broker processing of the ZTF public stream. We perform follow-up observations at the ANU 2.3m telescope in Australia and obtain 92 spectroscopic classified events that are incorporated in our training set. We show that our follow-up strategy yields a training set that, with 25% less spectra, improves classification metrics when compared to publicly reported spectra. Our strategy selects in average fainter events and, not only supernovae types, but also microlensing events and flaring stars which are usually not incorporated on training sets. Our results confirm the effectiveness of active learning strategies to construct optimal training samples for astronomical classifiers. With the Rubin Observatory LSST soon online, we propose improvements to obtain earlier candidates and optimise follow-up. This work paves the way to the deployment of real-time AL follow-up strategies in the era of large surveys.​
ELEPHANT: ExtragaLactic alErt Pipeline for Hostless AstroNomical Transients
Pessi et al., 2024
​
We present the ExtragaLactic alErt Pipeline for Hostless AstroNomical Transients (ELEPHANT), a framework for filtering hostless transients in astronomical data streams. We used Fink to access all the ZTF alerts produced between January/2022 and December/2023, selecting only those associated with extragalactic transients. We then processed the associated stamps using a sequence of image analysis techniques to retrieve hostless candidates. We find that less than 2% of all analyzed transients are potentially hostless. Among them, approximately 10% have a spectroscopic class reported on TNS, with Type Ia supernova being the most common class, followed by SLSN. Among the hostless candidates retrieved by our pipeline, there was SN 2018ibb, which has been proposed to be a PISN candidate; and SN 2022ann, one of only five known SNe Icn. When no class is reported on TNS, the dominant classes are QSO and SN candidates, the former obtained from SIMBAD and the latter inferred using the Fink ML classifier. ELEPHANT represents an effective strategy to filter extragalactic events within large and complex astronomical alert streams. There are many applications for which this pipeline will be useful, ranging from transient selection for follow-up to studies of transient environments.